Quantifying Ataxia
Ataxia patients lack specialist access. Using US clinic videos, our model predicts Ataxia risk and severity with high accuracy.
Ataxia is a neurodegenerative disease that surfaces as difficulty with motor control, such as walking imbalance. Many patients with Ataxia do not have easy access to neurologists – especially those living in remote localities and developing/ underdeveloped countries. In a multi-year collaboration effort with READISCA, we collected a video dataset of ataxia diagnosed and control subjects from 11 clinics located in 8 different states across the United States. The dataset contains 150 fully anonymized 6 seconds long videos from 89 unique study participants (24 control, 65 diagnosed) performing gait task.
Furthermore, we developed a computer vision and machine learning pipeline to identify, track, and separate participants from complex surroundings and predict their risk and severity of Spinocerebellar Ataxia. Ataxia risk-prediction model achieves 83.06% accuracy and an 80.23% F1 score. Ataxia severity-assessment model achieves MAE score of 0.6225 and a Pearson’s correlation coefficient score of 0.7268. Our model performs competitively while tested on clinics completely unseen during training. Our feature importance analysis shows the model automatically picks up traits that are consistent with established clinical knowledge.
The code and dataset:
https://github.com/ROC-HCI/Automated-Ataxia-Gait
Publications:
Rahman, M. Hasan, M. S. Islam, T. Olubajo, J. Thaker, A. Abdelkader, P. Yang, H. Paulson, G. Oz, A. Durr, T. Klockgether, T. Ashizawa, E. Hoque, Auto-Gait: Automatic Ataxia Risk Assessment with Computer Vision from Gait Task Videos, Proceedings of ACM on Interactive, Mobile, Wearable, and Ubiquitous Computing (IMWUT), 7(1), 1-19.
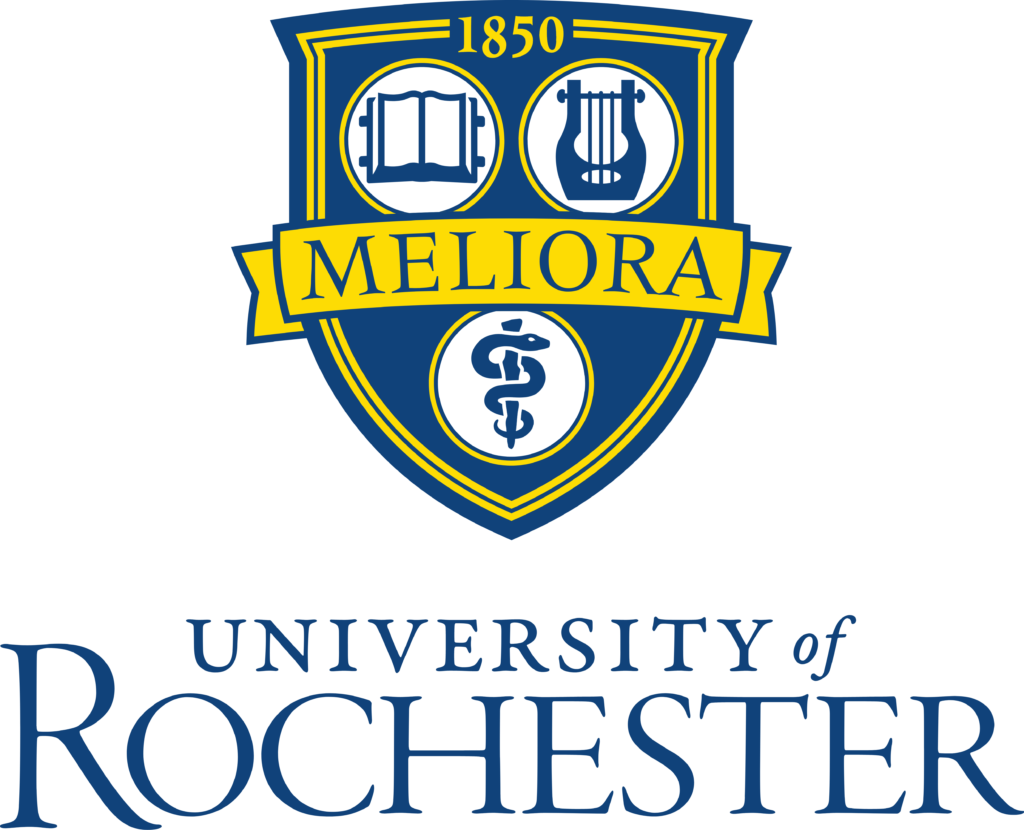
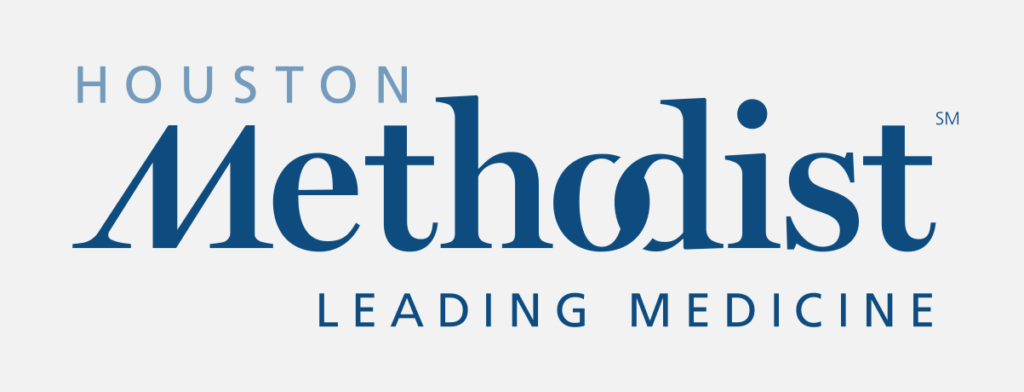
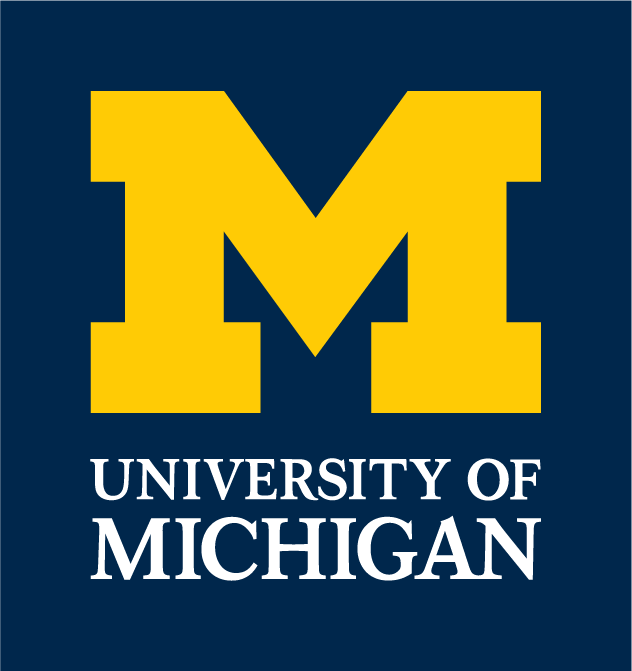
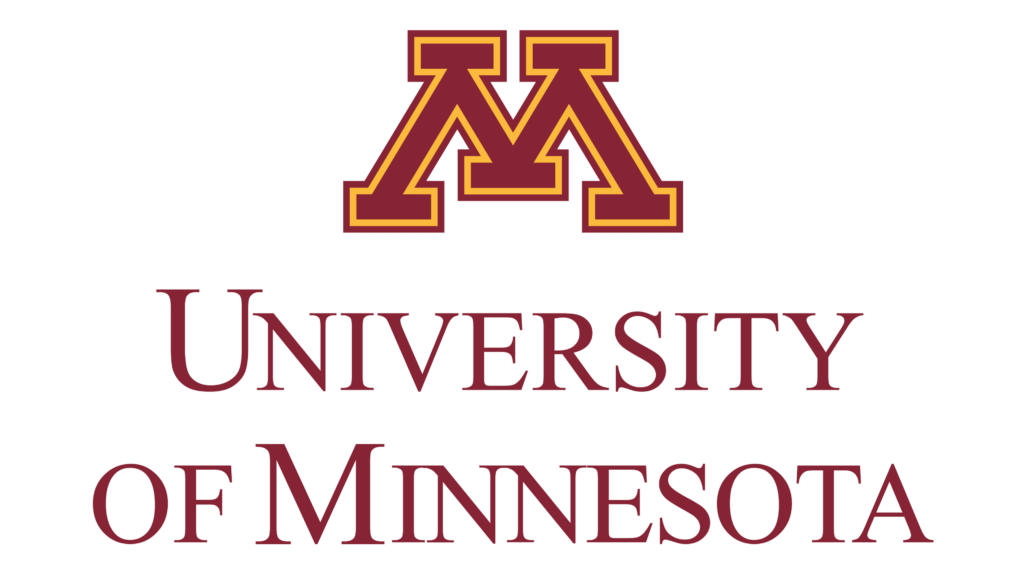

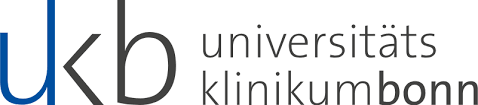
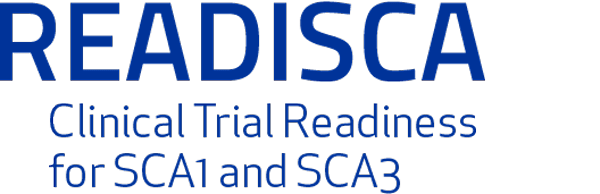
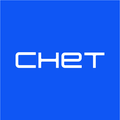